HIT Perspectives
Subscribe
HIT Perspectives – August 2023
Unleashing AI in Healthcare: Transformations, Triumphs, and Turmoil
By Ed Daniels, Senior Consultant
Quick Summary
- The use of AI — especially large LLMs — has expanded at an unprecedented pace since December 2022 and this growth will continue for the foreseeable future.
- The most jarring accomplishments have been the improvements in ability to understand and respond in human languages, but understanding and generating images, videos, computer code and mathematical expressions is no less astounding.
- These advances in AI promise many new beneficial scientific discoveries, robotic tools and software-driven services. They also bring many new threats and dangers to human well-being.
- All sectors of the healthcare industry are being impacted by these advances. For healthcare, the benefits of AI are much needed and therefore amplified due to the complex challenges in improving diagnosis and treatment of disease as well as enhanced health, quality of life and longevity. But threats are also amplified because of the potential for more misinformation, inequality/bias, privacy breaches and errors in diagnostic and treatment processes.
- Billions of dollars are being invested in attempts to control, align and error-proof new AI algorithms, but these models are complex, challenging and currently not reliable. As the AMA suggests, these AI tools should be used to “augment intelligence” with physicians and other qualified healthcare practitioners serving as the final authority.
Artificial Intelligence (AI), a branch of computer science inspired by biology, empowers systems to perform tasks that typically require human intelligence. Learning, adapting to new information, understanding natural language, recognizing patterns, problem solving, and decision making are all within its capabilities. Large language models (LLMs), a specialized form of AI, have revolutionized the field, enabling seamless communication in human languages and processing vast amounts of data from the Internet, libraries, scientific literature and more.
OpenAI's GPT 3.5, GPT 4, ChatGPT, and Google's Bard are among the most remarkable AI advancements. These models can generate essays, answer questions, translate languages and even create poetry. The term "GPT" stands for generative pre-trained transformer, highlighting the underlying technical architecture of these models.
Beyond text, generative AI extends its reach to image and video content creation based on textual prompts. Notable tools like Midjourney and DALL-E fall into this category. The "generative" aspect denotes their ability to produce novel outputs, elevating them beyond mere classification or decision making based on inputs.
As AI continues to evolve, the future of healthcare holds immense potential for transformation. The combined power of AI technologies like ChatGPT promises to revolutionize patient care, diagnostics and medical research, reshaping the landscape of healthcare as we know it.
Historical Use of AI in Healthcare
The healthcare industry has long employed AI for population health management. Organizations like insurance companies and accountable care organizations leverage AI's predictive analytics to analyze medical records and claims data using regression and machine learning models including neural networks.
AI's potential shines in its ability to process vast amounts of clinical data. From lab results and imaging data to patient demographics, AI algorithms identify patterns and correlations. These insights enable accurate predictions of disease progression, identification of high-risk patients and personalized treatment plans.
By analyzing patient data, including medical history and risk factors, AI assists doctors in making informed decisions. It identifies individuals at risk of developing certain conditions and recommends preventive measures to improve patient outcomes.
AI goes beyond human capabilities, detecting subtle patterns and social determinants of health that might elude healthcare providers. A notable example is NYUTron, an AI model at NYU Langone Health System predicting readmission probability, in-hospital mortality, comorbidity index, length of stay and insurance denial.1
AI's presence in healthcare continues to evolve, promising transformative impacts on patient care and medical decision making.
Recent Advances in AI Use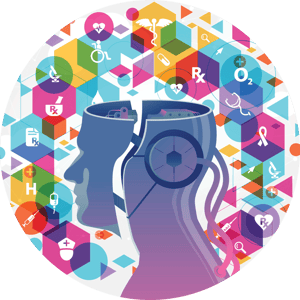
AI with new language capabilities analyzes unstructured data (e.g., clinical notes, medical literature) to aid diagnosis and discover symptom-disease associations.
Med-PaLM 2 is an LLM designed by Google specifically to respond to medical questions. Med-PaLM 2 performs expertly, scoring 85.4% on the US Medical Licensing Exam. It responds to medical questions, while its parent, PaLM 2, handles advanced reasoning, coding, advanced mathematical calculations and multilingual tasks.
According to public announcement, this April, Microsoft and Epic Systems will utilize OpenAI's GPT-4 AI language model in healthcare. GPT-4 will draft message responses for patients and analyze medical records, identifying trends. It will also power natural language queries with Epic's SlicerDicer. Separately, Mayo Clinic uses Google's "Enterprise Search on Generative AI App Builder" service to review vast amounts of internal data efficiently.2
AI is also being used in drug discovery to assist with drug design, chemical synthesis, drug screening, polypharmacology and drug repurposing. AI can provide access to new biology and improved or novel chemistry. This can result in higher success rates, quicker and cheaper discovery processes and drug discovery cost reductions of as much as 70%.3
One dramatic accomplishment was realized by AlphaFold, a deep learning system developed by Google’s DeepMind. AlphaFold can predict the three-dimensional (3-D) structure of a protein from its amino acid sequence. This tool helps researchers understand how proteins work and how they can be targeted with drugs.
In a different medical realm, AI models excel at rapidly detecting and classifying abnormalities in radiographs, computed tomography scans, magnetic resonance imaging, and analyzing electrocardiograms, pathology slides and ophthalmic images. For example, HeartBEiT, a vision-based transformer model, achieved higher diagnostic performance by highlighting biologically relevant regions of the electrocardiogram, after training on 8.5 million ECGs.4
AI may also revolutionize surgical procedures by integrating with artificial reality and virtual reality for enhanced navigation, providing real-time 3-D visualizations to improve precision and reduce complications.5 Analyzing surgical videos allows recognition of distinct phases, aiding surgeon training and procedure development.6 Additionally, AI assists in preoperative planning, predicting outcomes and identifying risks from patient data. In the future, AI could integrate with robotic systems for precise surgery and reduced surgeon fatigue.7
AI can play a significant role in analyzing genetic and genomic data to suggest new ways to diagnose and treat disease. For instance, machine learning algorithms can be used to analyze genetic data to identify mutations or variations associated with specific diseases. This can help in the early detection of diseases and development of personalized treatment plans based on a patient's genetic makeup.
In the field of genomics, AI can be used to analyze large-scale genomic data to understand how different genes interact with each other and how these interactions contribute to disease. This can lead to the discovery of new disease pathways and the development of novel therapeutic strategies. AI can help in the annotation and interpretation of genomic data, which is a significant challenge given the vast amount of data generated by genomic sequencing technologies.
AI can enhance the training of medical students and students in medical fields by providing personalized, interactive and scalable learning experiences. Serving as an intelligent tutoring system, AI can adapt to the individual learning pace and style of each student, providing personalized feedback and identifying areas that need improvement.
AI can be used to create realistic virtual patients and simulations for hands-on practice, allowing students to gain practical experience without risk. AI can analyze enormous amounts of educational data to identify effective teaching strategies and areas where curricula may need to be adjusted. Machine learning algorithms can also be used to predict student performance and identify students who may need additional support. Overall, AI has the potential to revolutionize medical education by making it more personalized, efficient and effective.
Future Developments
AI-driven conversational agents known as “bots” have been undergoing development and improvements for many years, and will increasingly be able to represent companies, physician practices and hospitals in natural language text, audio and video conversations. These conversations with customers and patients will better manage the logistics of appointment scheduling, prescription refill requests and other routine clinical and administrative tasks.
The future of LLMs across all industries, including healthcare, will see a proliferation of bots specialized for specific purposes. There will be customer service bots, call center bots, appointment-setting bots, clinical advice bots, mental health counselor bots and just friend or companion bots. You might say, “We already have those,” but these new bots based on LLMs will be far more capable. They will be able to text, email and speak on many specialized topics in 100 different languages. The primary obstacle currently is training and constraining LLMs to perform a particular task, only speaking the company-approved truth and never straying to converse on non-sanctioned topics.
The Dark Side of AI
The rise of LLMs and generative AI brings amazing potential but also introduces new risks. Surprisingly, GPT 3.5, GPT 4, and Google's Bard can lie and hallucinate, raising concerns in medical settings where patients might be misled.
Developers of healthcare AI tools must be vigilant and root out biases in AI models. For example, a model trained on datasets that underrepresent certain patient groups, like gender and ethnic minorities, might mistakenly propagate deeply rooted societal biases, amplifying inequalities, misdiagnosing certain diseases, and proposing ineffective or damaging treatments.8
Privacy protection is also crucial when handling personal health information (PHI) within LLMs. Training on vast data from diverse sources makes it challenging to ensure data do not include PHI or false PHI in responses. Research and development are underway to address this issue, but AI is being used in healthcare now.
The inner workings of AI models, including LLMs, are not fully understood due to their ability to develop independent self-improvement strategies. This creates the "explainability" or "black box" problem.10 Developers are exploring various approaches, from asking the LLM directly to fact-checking and incorporating explanations into the model's fabric. Additionally, researchers are probing the model's neural network to gain insights into its processes.
Researchers are actively addressing these concerns, seeking ways to incorporate fact-checking and explanation into AI models. Probing the neural network's processes offers insights into how AI handles questions and answers.
These complexities lead to cautious adoption by healthcare providers and calls for new layers of regulation and oversight. The quest for transparency and reliability in AI recommendations continues to shape the future of AI in healthcare.
Current and Future AI Regulation
The US Health and Human Services Department's proposed rule, published on April 18, 2023, delves into AI and associated clinical decision support algorithms. The rule suggests adding a "Decision Support Interventions" certification criterion to keep up with healthcare decision-making software advances. The proposed regulation is crucial for companies developing or considering AI-enabled electronic health record software.
Current laws and regulations in the United States (US), Europe and globally apply to new AI tools, such as Trusted Exchange Framework & Common Agreement, 21st Century Cures and General Data Protection Regulation, focusing on data privacy and security. However, these legacy regulations may not fully address the latest AI technology. Congress considers bipartisan AI bills9 and other areas where additional regulation may be needed. While evaluating legislative action related to AI, Congress aims to understand the evolving technology's implications, particularly in healthcare. The White House "Blueprint for an AI Bill of Rights" highlights the challenges posed to democracy by technology and data misuse. Chief executive officers from Google and Microsoft, now in healthcare, met with President Biden and Vice President Harris to discuss AI's merits and risks, as well as safeguarding society.
There is a growing recognition of concerns regarding the possible misuse or unintended negative effects of AI. Consequently, various initiatives have been undertaken to address these concerns and establish standards. For instance, the US National Institute of Standards and Technology has launched an initiative that involves organizing workshops and engaging in discussions with both the public and private sectors. The aim is to develop federal standards that serve as the foundation for creating reliable, robust and trustworthy AI systems. A number of Executive Orders have also been issued in this regard.
Furthermore, state lawmakers have begun to acknowledge the benefits and challenges posed by AI. To better understand its impact and explore the role of policymakers, an increasing number of measures are being introduced at the state level. These measures focus on studying the effects of AI and algorithms, as well as considering potential avenues for policy development in this field. However, not all of the measures introduced are tied specifically to health care.
Public opinion about AI varies, ranging from excited anticipation to fear and avoidance. These intersecting forces are creating a dynamic interplay between public opinion and legislative decision making. The debate is being fueled by AI’s own tech leaders, who are publicly calling for additional regulation of their own industry.
Conclusion
For Point-of-Care Partners’ (POCP) clients, this is a pivotal time. These AI and, specifically, LLM tools hold tremendous promise for increased efficiency, improved clinical results, better customer service and increased profits. Yet knowing where to go next in pursuit of this technology is difficult to determine as the technological and regulatory environments change rapidly. While this is happening, patients and consumers are increasingly concerned about the impact of AI on their privacy and security.
POCP believes it is essential for companies to get involved and understand this AI revolution. What are the opportunities and what are the dangers? Just keeping on top of these changes requires a certain level of investment and experimentation. The risks are significant, but they cannot be avoided by staying out of it. They can only be minimized by being careful about where to invest and what to implement when.
If we can help you navigate these technologies that hold so much promise yet so much risk, let us know. We would be pleased to help you understand the potential for your own business and discover what other companies in your market are doing or considering.
References:
1.) https://www.nature.com/articles/s41586-023-06160-y and "Malnutrition and its impact on cost of hospitalization, length of stay, readmission and 3-year mortality" by Su Chi Lim et al https://www.researchgate.net/publication/235370394_Reply_-_Malnutrition_and_its_impact_on_cost_of_hospitalization_length_of_stay_readmission_and_3-year_mortality
3.) According to the “Insider Intelligence’ AI in Drug Discovery and Development report” https://www.insiderintelligence.com/insights/ai-machine-learning-in-drug-discovery-development/
4.) https://www.nature.com/articles/s41746-023-00840-9
5.) Discussed in the paper by Hamid Ghaednia et al “Augmented and virtual reality in spine surgery, current applications and future potentials” https://einstein.elsevierpure.com/en/publications/augmented-and-virtual-reality-in-spine-surgery-current-applicatio
6) Suggested in the paper by Shoichi Nishio et al. “Real-Time Orthopedic Surgery Procedure Recognition Method with Video Images from Smart Glasses Using Convolutional Neural Network” https://www.researchgate.net/publication/330475648_Real-Time_Orthopedic_Surgery_Procedure_Recognition_Method_with_Video_Images_from_Smart_Glasses_Using_Convolutional_Neural_Network
7.) https://doi.org/10.1016/j.spinee.2021.03.018 “Augmented and virtual reality in spine surgery, current applications and future potentials”
9.) https://www.politico.eu/article/artificial-general-intelligence-policy-public-technology/ “AI policy needs to bring the public with it”